Did you know that whenever you browse the internet, you encounter the work of Generative AI? Be it the news articles you read, the images you see, or even the music you listen to, AI has quietly become a creative force in our lives. But here’s the catch: While generative AI has transformed industries, it’s not without its risks.
In this article, we take a deep dive into ‘The Biggest Risks of Generative AI’. ‘These are not mere possibilities; They are concrete threats that demand our attention. So, let’s peel back the layers of this technological marvel and uncover the challenges that could reshape our future.
Bias and Discrimination of Generative AI
Bias and Discrimination Generative AI, short for Generative Adversarial Networks (GAN), is a subset of artificial intelligence that excels at autonomously generating content such as images, text, audio, and video. It works on the principle of two neural networks – one generating content and the other evaluating it – that work to improve the output over time.
However, generative AI is not immune to the imperfections of the data it is trained on. It may inadvertently inherit biases present in its training data. Imagine that the training data primarily consists of certain demographics, locations, or viewpoints. AI models will naturally lean toward replicating these biases in the content they generate.
This bias problem extends to racial, gender, and cultural biases, which can perpetuate stereotypes and disparities in AI-generated outputs. To illustrate this, consider the case of AI-powered language models that inadvertently produce sexist or racist content. For example, AI chatbot descriptions reinforce gender stereotypes or AI-generated text that displays racial biases.
These real-world examples are a stark reminder of the potential harm that generative AI can deliver if not rigorously monitored and controlled.

Increasing Social Engineering Attacks on Generative AI
Increasing Social Engineering Attacks Generative AI, with its ability to mimic human-like behaviors and create hyper-realistic content, has become a powerful weapon in the hands of malicious actors, significantly increasing social engineering attacks.
Think of it as giving a master counterfeiter access to a printing press. Imagine this: AI-powered chatbots, indistinguishable from humans, craft messages that trick individuals into revealing sensitive information or clicking on malicious links. These chatbots can impersonate trusted entities, making them incredibly difficult to distinguish from fakes.
Video-based generic AI takes things a step further, supercharging deep fake attacks. Facial recognition security measures fail in the face of this technology. Bad actors can easily carry out spoofing attacks by impersonating company employees. Text-based generative AI, like ChatGPT, can generate highly personalized emails, enabling spear phishing on an unprecedented scale.
To add complexity, attackers are increasingly using audio-generating models to create fake audio clips. Imagine you are receiving a voice message from your CEO instructing you to take a specific action, but you realize it is a cleverly crafted fake. The threats are real and growing.
Malicious campaigns exploiting generative AI have been reported across a variety of distribution channels, from fake social media pages to browser extensions. These attacks aim to steal session cookies, launch SEO poisoning attacks, and impersonate trusted sources, leaving individuals and organizations vulnerable to manipulation.
The Creation of Sophisticated Malware of Generative AI
The creation of a sophisticated malware-generative film is not simply a tool for creating art or content; It can also be vulnerable to robotic malware that is adapted and evolved, making it a tough challenge for cybersecurity. Traditionally, malware authors had to avoid identifying their code as nominal or rely on simple actors.
But with flexible architectures, hackers can train systems to generate polymorphic malware – malware that retains its original structure, changing its code and appearance as it is stored. Like resizing is detected to avoid malware.
There is now access to tools like WormJPT and FraudJPT, which ChatJDP has, which are like ChatJDP’s Citroën Cruz brother, which study specifically on malware-data. These hotel-operated devices can take advantage of drylice, launch Business Email Compromise (BEC) attacks and quickly become malware variants.
Result? A cyber security landscape filled with ever-increasing intelligence and sophistication. The big risk of data analysis and identity theft is its imagination: custom-architected models, those clever digital creations, trained and grown by the information you provide.
But here’s the problem: Many businesses are still experimenting with host systems without strong controls in place for security. Education-related information. This means that user, incognito, owner and trust information is shared with Masala chatbots. Research conducted by data security company Cyberhaven revealed that ChatGPT has 11% data trust stuck to it.
Bypassing Traditional Security Protections of Generative AI
It’s like accidentally telling your company secrets to a chatbot. This uncontrolled use of generative AI tools increases the risk of data breaches and identity theft to dangerous levels. Picture this: a data leak incident that made headlines earlier this year. It exploited a vulnerability in the source code of ChatGPT, resulting in a breach of sensitive data. A vulnerability in the Redis memory database used by OpenAI allowed unauthorized actors to gain access to users’ chat histories. The incident exposed the personal and payment data of approximately 1.2% of active ChatGPT Plus customers. Examples like this are a goldmine for malicious actors. Sensitive data can be stored, accessed or misused, potentially fueling targeted ransomware or malware attacks that could paralyze business operations. Bypassing traditional security protections Now, imagine hackers armed with generic AI algorithms that can detect and exploit vulnerabilities in security systems. These algorithms are like cyber bloodhounds, but on steroids.
They easily outperform traditional protections such as signature-based detection and rule-based filters. This AI-powered technology streamlines the process of finding and exploiting vulnerabilities, making it easier for malicious actors. They can quickly pinpoint and target weak points in the system or software, reducing their manual efforts. Result?
Organizations find themselves at the mercy of attackers, vulnerable to data breaches, unauthorized access and other security nightmares. Model Manipulation and Data Poisoning
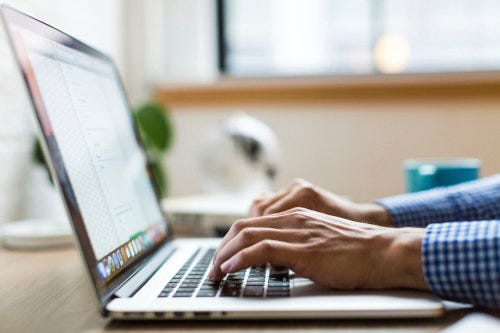
Model Manipulation and Data Poisoning of Generative AI
Imagine this: adversaries, digital mischief-makers, are deliberately tampering with the training data of generative AI models. It’s like someone adding harmful ingredients to a recipe, except the result is poisonous.
These opponents have some tricks up their sleeves. They introduce vulnerabilities, backdoors or biases into the training data, undermining the safety, effectiveness and ethical behavior of AI models. It’s like infiltrating an army of spies, ready to spread chaos from within. Consider a recent incident discovered by ethical hackers: an instant injection attack targeting users of ChatGPT.
This covert attack modifies chatbot replies and exposes the user’s sensitive chat data to malicious third parties. twist? It could be designed to continuously influence all future answers like a digital parasite. This is data poisoning in action, and a powerful weapon in the wrong hands. But why is data poisoning so dangerous? Because it corrupts the very essence of generative.
If a model is exposed to toxic data during training, it can start producing harmful, biased, or downright misleading outputs. Think of it as an AI artist tainted by a malicious motivation, who is creating paintings that spread misinformation, perpetuate stereotypes and discrimination, and tarnish reputations.
In real-world applications, this can cause a number of problems, from damaging a company’s reputation to facilitating the spread of dangerous misinformation. Data poisoning is not just an isolated incident; It is a ticking time bomb that demands vigilance and robust security in our increasingly AI-driven world.
The risks we highlight, from bias and misinformation to data breaches and model manipulation, are not hypothetical scenarios; They are real, current and evolving. However, they do not need to define our AI-powered future. And that’s why, our journey through the world of Generative AI is not over yet. This is a journey that requires all of us to be informed, engaged and active. The decisions we make today will shape the future of AI for generations to come.
stay tuned for the latest information about tech and innovations at Technofash.